The Psychology of AI Interfaces: Building Trust Through UX
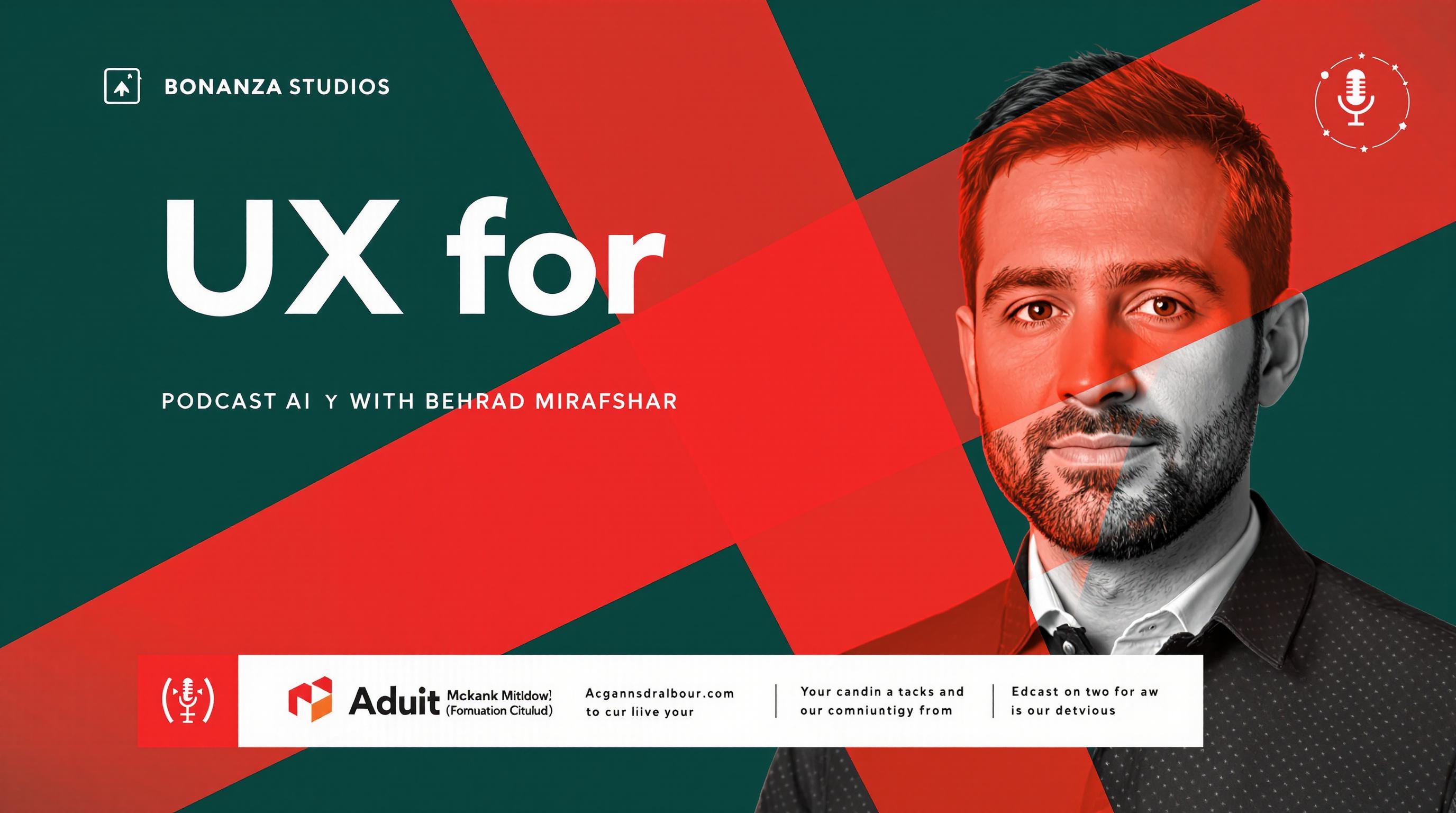
How do you build trust in AI? Transparency and user control are key. Research shows that trust in AI systems varies by region, with EU users being more skeptical due to privacy concerns like GDPR, while U.S. users are generally more accepting. To bridge this gap, AI systems need clear communication, user control features, and region-specific designs.
Key Takeaways:
- Transparency: Real-time confidence indicators and clear explanations help users understand AI decisions.
- User Control: Options like opt-outs and adjustment features reduce resistance to AI.
- Regional Design: Tailored interfaces that align with local preferences build trust faster.
Quick Comparison of Trust in AI: EU vs. U.S.
Aspect | EU | U.S. |
---|---|---|
Trust Level | Lower (43% less trust) | Higher |
Focus Areas | Privacy, transparency (GDPR) | Performance, usability |
Preferred Features | Explicit consent, oversight | Simplicity, efficiency |
To build better AI interfaces, focus on transparency, user control, and localized designs. These strategies not only improve user confidence but also ensure compliance with regulations like GDPR and the AI Act.
Designing for Trust (PAIR UX Symposium 2018)
Trust Factors in AI Systems
Building user trust in AI systems depends heavily on their performance and openness. Studies reveal that trust in AI differs greatly from how we trust other people.
System Capability and Intent
Users judge AI systems based on how reliably they perform and whether they deliver value. These systems need to show they can handle tasks effectively while keeping the user's needs in mind. When errors occur, providing clear explanations can help rebuild trust.
"The key to evaluating trustworthy AI is whether AI does what it claims to do." – Schwartz et al.
Additionally, open and straightforward communication strengthens user confidence in the system.
Clear System Communication
Features like real-time confidence indicators help explain how decisions are made and ensure compliance with transparency standards, such as AI Act Article 13. For example, in 2021, Aoki found that informing users about the role of human oversight in AI-powered healthcare tools significantly increased trust. This shows the importance of maintaining a balance between transparency and usability.
User Choice and Control
Beyond performance and communication, giving users control over AI systems is crucial for building trust. Research by Dietvorst et al. (2018) revealed that even small opportunities for users to adjust algorithmic outputs can significantly reduce their resistance to automated systems.
Options like opt-out features, adjustment controls, and visible oversight signals not only empower users but also align with GDPR Article 22. This is especially impactful in areas like healthcare, where being transparent about human supervision can greatly enhance trust in AI solutions.
Trust-Building UX Methods
Good UX design plays a key role in building user trust in AI systems by focusing on transparency and user control.
System Confidence Displays
Real-time confidence indicators can help users understand how AI systems make decisions. For example, color-coded signals showing levels of certainty make the decision-making process clearer. In areas like healthcare, interfaces that display confidence levels alongside key factors used in assessments allow users to better grasp the reasoning behind recommendations. This level of clarity is especially important in high-stakes scenarios.
User Control Features
Beyond transparency, giving users control over AI systems can improve engagement and trust. Features like override options, feedback tools, and behavior controls allow users to influence how the AI operates. This reduces resistance to algorithms and fosters a sense of collaboration. Yngvi Karlson, Co-Founder of Kin, shared:
"When people know they can give feedback on AI systems like AI companions, can see it is being listened to, and have a way to confirm its impact, their relationship with not just the AI applications but the entire AI governance structure suddenly becomes two-way."
Regional UX Adjustments
Tailoring UX design to align with regional cultural preferences strengthens trust and encourages user interaction. Localized designs that reflect cultural norms, along with standardized trust icons and clear explanations, help users quickly understand AI recommendations. In areas where AI skepticism is higher, practices like transparent data usage and explicit consent processes further reinforce trust.
sbb-itb-e464e9c
Brain Science in AI Design
Blending brain science with UX design enhances user trust by emphasizing visible trust signals and personalized design elements. Recent studies using neuroimaging tools like EEG, fMRI, and fNIRS have uncovered specific brain activity patterns during user interactions with AI systems. These findings provide a foundation for improving how users respond to AI, as explained below.
Trust Icons and Processing Speed
Using standardized trust indicators has been shown to improve how quickly users process information. Research reveals that interfaces with these trust icons enable users to process AI recommendations 22% faster compared to those without them. Lower galvanic skin responses also indicate that clear trust signals help reduce stress and make users feel more at ease. By speeding up processing and reducing stress, well-designed trust elements naturally align with how people think and build confidence in the system.
Key Design Elements for Trust
Studies highlight two critical factors for building trust: competence and warmth. Effective designs demonstrate competence through features like real-time performance metrics, safety notifications, and robustness. Warmth is conveyed through privacy assurances, transparent decision-making explanations, and empathetic interactions.
Neuroimaging research supports the idea that AI interfaces should:
- Reduce perceived uncertainties by presenting clear, concise information
- Provide straightforward explanations for decision-making processes
- Use trust signals that align with how users naturally process information
While adding human-like features can increase trust, they must be used carefully to maintain the system’s credibility. These neurological insights reinforce previous UX strategies, showing that designs informed by brain science are crucial for creating reliable and trustworthy AI systems.
Trust Testing Tools
After exploring trust-building UX methods, the next step is testing these features to ensure they work effectively. Testing tools and frameworks combine ethical guidelines with practical measures to create AI interfaces that are both trustworthy and compliant.
SAP AI Ethics Integration
The SAP AI Ethics Toolkit offers a structured approach to testing trust signals while adhering to regulations like the EU AI Act. This toolkit focuses on human-centered design and includes important trust-building features:
- Real-time AI notifications: Alerts that inform users when they’re interacting with AI-generated content.
- Risk-based disclosures: Detailed information provided for higher-risk AI applications.
- Visual trust signals: Standardized icons that identify AI-powered actions.
"SAP Business AI is designed for people to get their best work done, valuing human oversight and agency." - SAP
This integration ensures transparency and user privacy by monitoring regulatory changes, enforcing standardized trust indicators, and keeping security measures up to date.
Trust Element Testing
Testing trust in AI interfaces involves several measurement methods. A well-rounded framework might include:
Measurement Type | Metrics | Implementation |
---|---|---|
Self-Reporting | User questionnaires, trust scales | Post-interaction surveys |
Behavioral Analysis | Decision time, compliance rate, delegation patterns | Automated tracking |
Physiological Indicators | Skin conductance, eye tracking | Real-time monitoring |
These methods allow for quick updates and improvements to trust features. Studies show that combining self-reporting, behavioral analysis, and physiological data provides accurate insights, helping refine trust signals in real-time.
Conclusion: Building Better AI UX
Creating trust in AI systems requires a deep understanding of both technical and human factors. Research highlights that trust levels can vary significantly across regions and demographics, making tailored interface strategies a critical component. As discussed earlier, prioritizing transparency, user control, and region-specific design are key to building trust.
Standardized trust indicators, when aligned with cultural and demographic insights, have shown positive results. For instance, studies indicate that users with university education often display greater trust in AI systems, emphasizing the need to consider user backgrounds during the design process.
Key Components of AI Interface Design
Trust Component | Implementation Strategy | Impact on Users |
---|---|---|
Transparency | Real-time confidence displays | Improves processing ease |
User Control | Always-available opt-outs | Increases engagement |
Cultural Design | Region-specific explanations | Addresses trust diversity |
"Trustworthy AI, defined as AI that is lawful, ethical, and robust"
– High-Level Expert Group on AI (AI HLEG)
To maintain and grow trust over time, regular evaluation is essential. The future of AI interface design will rely heavily on personalized and adaptable interaction strategies. By integrating user-specific characteristics into development while adhering to ethical standards, organizations can create interfaces that resonate with a wide range of users.
Ongoing testing and refinement are crucial. Combining user feedback, behavioral data, and physiological insights allows designers to fine-tune trust-building features. At the same time, compliance with changing regulations ensures systems remain reliable and user-focused.